QUANTUM-ENHANCED ARTIFICIAL INTELLIGENCE
Unification of quantum technology and AI is a two-way street of mutual aids. On one hand, creating quantum algorithms to address hard questions and interpreting noisy quantum data generated from a quantum processor (data packets contained in qubits) can be substantially improved with AI. Conversely, the ability of quantum algorithms to mitigate computing time complexity down to polynomial range can in return optimize and stabilize AI to efficiently confront with problems untraceable by classical computers. Together, there are lots of room for efficiency improvement.
Artificial intelligence (AI) needs quantum computing to attain indispensable progress towards the Artificial General Intelligence (AGI), a system capable of human-level thinking and machine consciousness. As the two technological transformations integrate into quantum artificial intelligence (QAI), the core value would be using quantum algorithms to enhance computational tasks within the perimeter of AI. At present, QAI is rather conceptual than practical because quantum computers that can tackle real-life problems do not yet exist. Expectantly, the very first challenge of initial practical studies would be the computation that enhanced machine learning algorithms impossible to undertake by the classical counterpart. For example, quantum computing can be used for rapid training of adaptive machine learning, optimizing algorithms, and reasoning under uncertainties.
Quantum Tech: By focusing on exploiting quantum computation for machine learning algorithms, there are four basic procedures to control and model artificial quantum systems. First, converting data to a multi-dimensional array of numbers called quantum tensors for further use. Second, choose suitable quantum neural network models to perform quantum processing, e.g., extracting information concealed in entangled states. Third, sampling to find average value. Samples from a classical distribution are the results from identical measurements on a quantum state. Now quantum data had been fully converted to classical data ready for the final step which is evaluating a classical neural networks model to find data correlations. Potential quantum algorithms that can be executed using a quantum computer to enhance AI are deep learning, decision making, searching, and game theory.
Direct Societal Impact: Improving AI: Identifying bias in a series of AI algorithms, computing power to process massive data to build AI systems as well as seamlessly integrate AIs into existing systems.
Collateral Advantages: Supporting IT systems: create layered security systems, identify security threats and breaches, improve codes, ensure quality, optimize servers, process unstructured data, strengthen cybersecurity. Virtual reality marketing: collect and analyze data, make automated decisions, and target ideal audiences. Other related application: modeling neuromorphic cognitive systems.
Alternative Quantum Technology: –
Aided System: Cloud computing, hybrid quantum-classical computing.
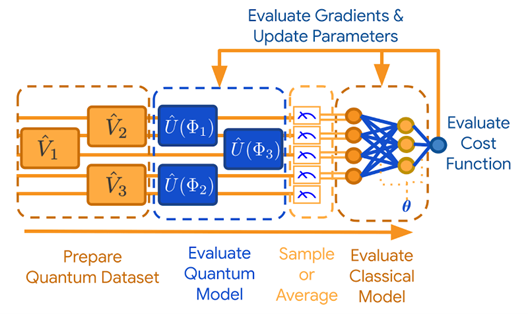